
[ad_1]
Whether or not you’re an NFL fanatic, an alumnus rooting in your alma mater or an excellent fan simply making an attempt to catch a glimpse of Taylor Swift, soccer season is likely one of the most enjoyable instances of the yr within the U.S.
And there’s no scarcity of how to take pleasure in it. Whereas hundreds of thousands of viewers will watch from the consolation of their couches or neighborhood bar, many others will trek to the stadium, generally in sub-zero temperatures, to see their favourite groups play – and, after all, tailgate within the car parking zone forward of the sport with different followers. Others could even need to hit the highway with the staff and journey to a brand new metropolis. However given followers have an entire season of video games to select from, they need assistance whittling down which of them to decide on.
Within the spirit of Databricks fixing our clients’ “hardest issues,” we needed to faucet into the facility of information and machine studying to assist NFL and faculty soccer followers predict how they will get essentially the most bang from their tailgating bucks.
On this weblog submit, we’ll stroll by means of how we used the Databricks Lakehouse Platform – together with Databricks AutoML and Databricks Assistant – with knowledge from our Databricks Market accomplice AccuWeather (who is aware of a factor or two about tailgating, being based mostly in Stage Faculty, PA – dwelling of the Penn State Nittany Lions) to reply the query: The place are the very best locations to tailgate the remainder of this season?
What we discovered
From November by means of December 2023, our mannequin pin-pointed 23 NFL video games out of the 117 complete that have been projected to have exemplary tailgating circumstances. We’re in a position to visualize these outcomes utilizing Databricks’ model new dashboarding instrument, known as Lakeview.
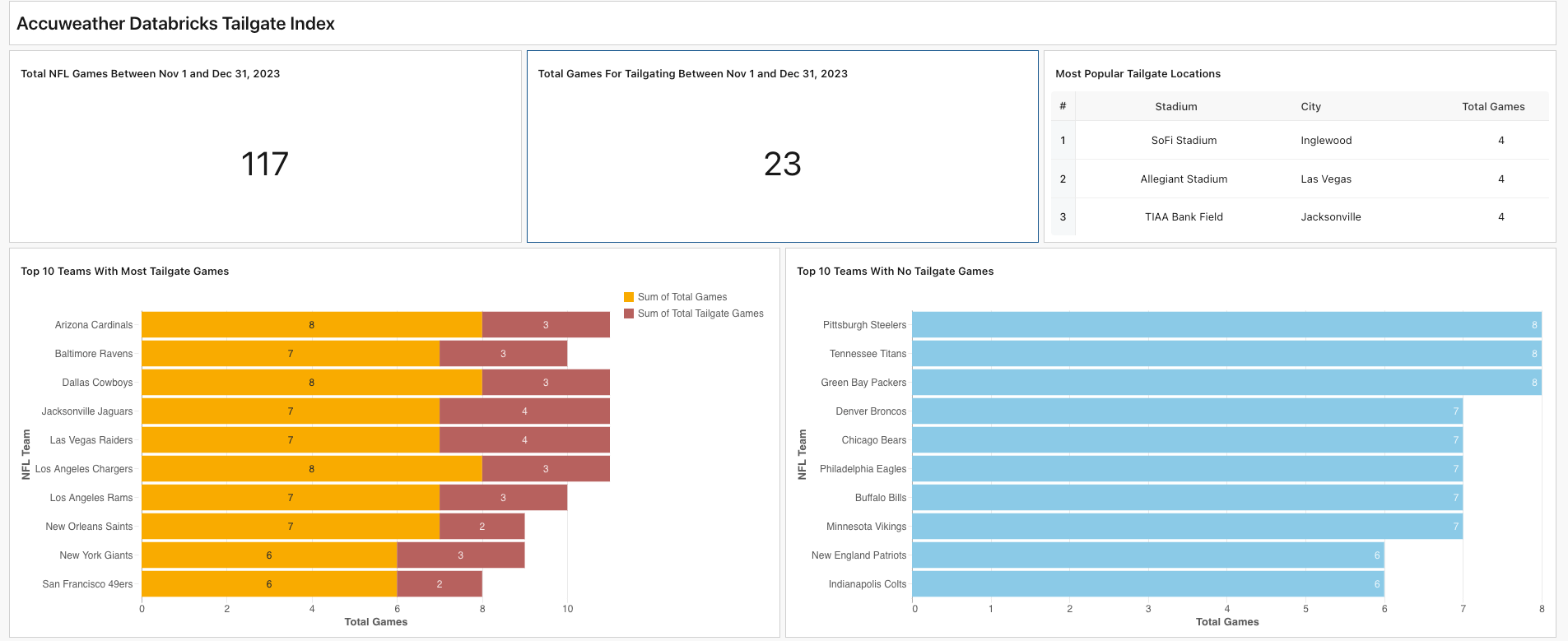
The stadiums with essentially the most “tailgate-able” video games have been SoFi Stadium in Inglewood, CA, Allegiant Stadium in Las Vegas, NV, and TIAA Financial institution Subject in Jacksonville, FL.
Much like the stadiums, it’s not too shocking that groups positioned in hotter areas are projected to have essentially the most best climate for his or her video games: the Arizona Cardinals, the Dallas Cowboys, the Jacksonville Jaguars and Las Vegas Raiders.
Conversely, followers of the groups with the fewest tailgate-able video games ought to get their heavy winter coats out of storage now – in the event that they haven’t already: the Pittsburgh Steelers, the Tennessee Titans, the Inexperienced Bay Packers, the Denver Broncos and the Chicago Bears. As we’ve seen earlier than, that’s unlikely to cease most of the devoted followers from trekking, probably in subzero temperatures, to the respective stadiums to tailgate. And that’s even with the tough begin to the season that lots of these groups are having.
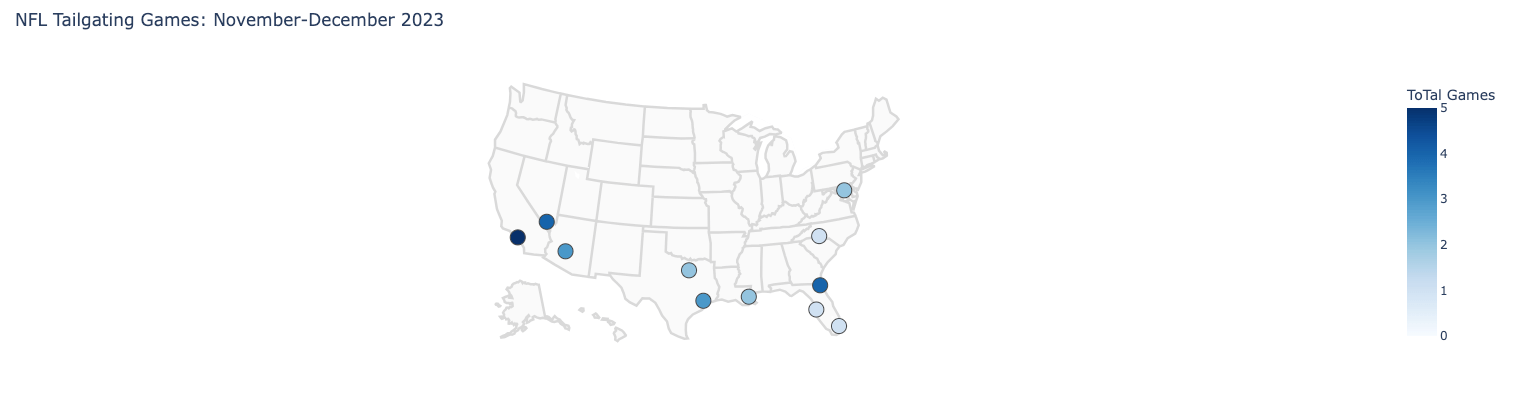
There have been a couple of surprises. Each the New York Giants/Jets and the Baltimore Ravens – not essentially groups from cities identified for his or her excellent climate circumstances in November and December – made it into the highest ten groups with essentially the most “tailgate-able” video games.
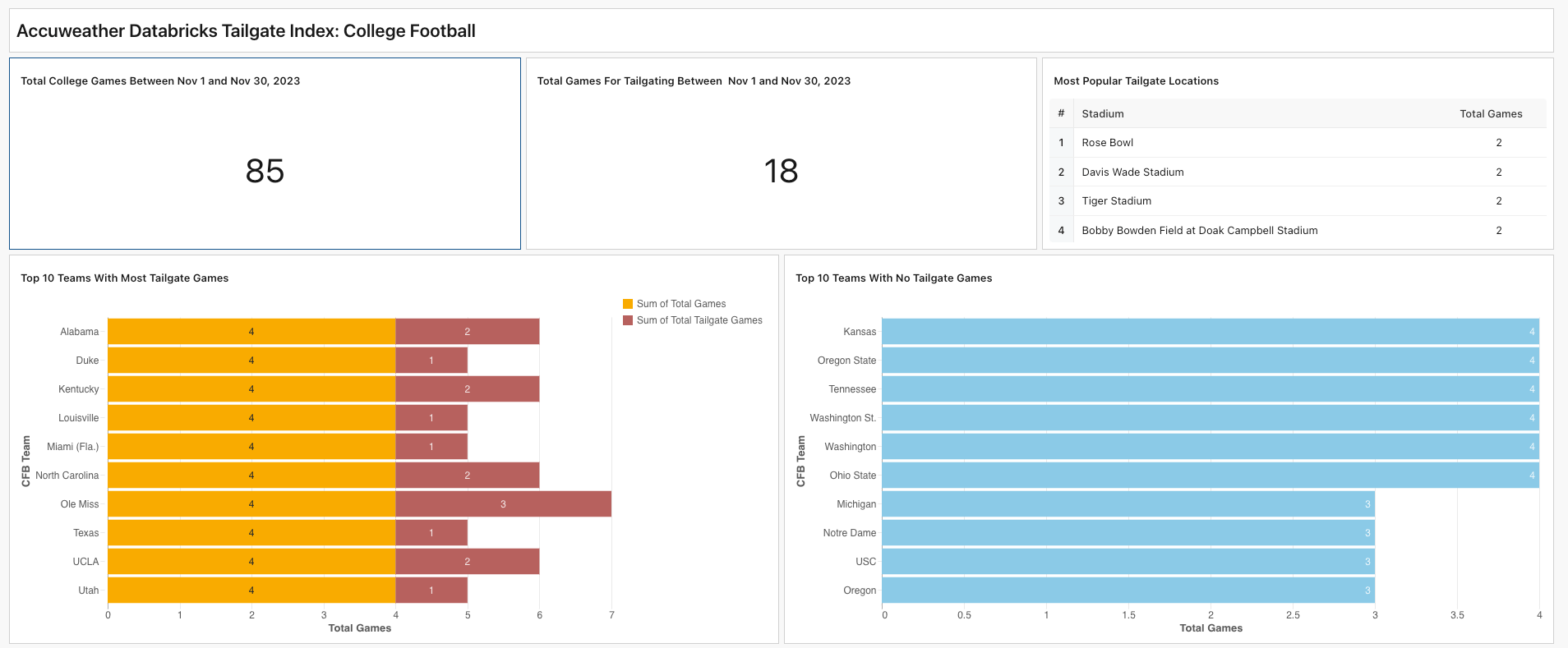
In the meantime, over the following few weeks, there are 18 faculty soccer video games that may doubtless show to be enticing tailgating choices. The highest 10 groups with essentially the most “tailgate-able” video games embody Alabama, Duke, Kentucky, Louisville and Miami. Conversely, the faculty groups whose followers ought to begin stocking up on scorching chocolate now embody Kansas, Oregon State, Tennessee and Washington St.
Why this issues
We get it, few enterprises are going to wish to know mission-critical tailgating info. However what should you did have to know when to inventory snow shovels, or when folks have been most probably to buy anti-frizz hair care merchandise? As we present with this use case, in relation to AI and ML, the tip utility is barely pretty much as good as the information and course of behind it.
With out gathering the appropriate knowledge, constructing the right mannequin, coaching it and verifying the outcomes, there isn’t any manner to make certain the mannequin is definitely performing as meant. By standardizing that course of on a single, unified knowledge platform, companies can begin to reap the advantages of AI and ML a lot sooner and with larger confidence within the outcomes.
What we are going to spotlight under is the step-by-step course of that we used to construct the Tailgate Index. However it’s simply repeatable for different use instances. For instance, change climate info with regional gross sales knowledge – just like the buyer dimension, location, trade, and so forth. – and the enterprise growth staff out of the blue has a chatbot that it will possibly use when evaluating potential new shoppers. As a substitute of querying the machine for the very best tailgate, salespeople may ask questions like: Inside this area, which companies are doubtless to purchase my product? Organizations can use climate knowledge and ML to foretell business-critical outcomes; as an illustration, a significant espresso chain could select to launch its pumpkin spice latte based mostly on colder-than-expected climate predictions.
Most significantly, Databricks helps to unlock the potential of information for everybody within the enterprise. With instruments like MLflow, it’s now potential for these with out knowledge science backgrounds to construct less complicated fashions – like classification, regression, and forecasting fashions. This democratization of ML and AI would be the catalyst that drives the effectivity good points so many companies are focusing on.
Our Strategy
The Databricks Lakehouse already serves as a unified platform to execute a large number of information and AI use instances, however some current options and enhancements that we’ll be strolling by means of made this undertaking simpler and sooner.
Getting knowledge, describing knowledge, knowledge abstract
As with each AI/ML undertaking, step one we settled on after determining the specified final result was getting the appropriate knowledge.
Working with Databricks accomplice AccuWeather, we have been in a position to use Delta Sharing and entry 4 years of climate info, spanning over 61 million information, within the Databricks Lakehouse in minutes. Along with cross-platform sharing of dwell knowledge, Delta Sharing allows organizations to find, consider and entry info shortly by means of the Databricks Market, the open market for knowledge, analytics, and AI.
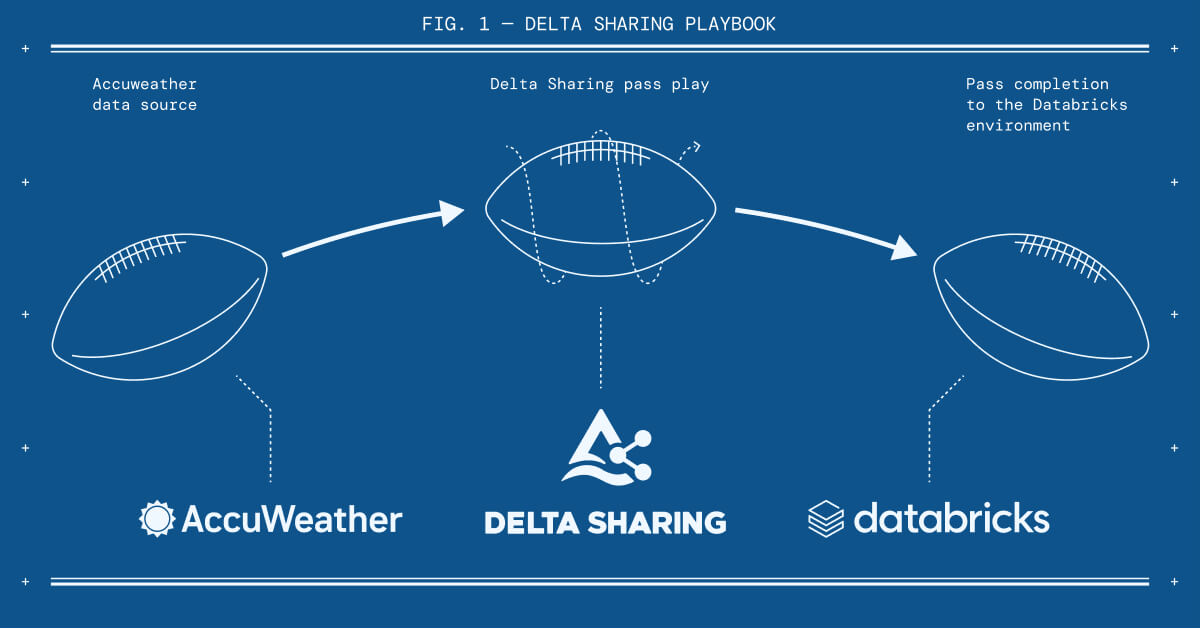
As soon as we had the information, we narrowed it all the way down to the timeframe of August to December and solely used days with soccer video games – Thursdays, Saturdays, Sundays and Mondays. That left us with 17 million.
When constructing ML fashions, it’s frequent to phase a portion of the coaching knowledge to validate the mannequin. Usually, it’s about an 80-20% break up between coaching and validation knowledge, respectively. On this occasion, we used 14 million information to coach the mannequin and three million to validate it.
These steps are essential, as they assist slim down the scope of knowledge the mannequin will likely be analyzing. In machine studying, the purpose is to remove as a lot pointless noise as potential. It didn’t make sense to coach our mannequin on previous info that wasn’t relevant to the end result we have been hoping to attain. And finally, the extra related the information that the mannequin is skilled on, the higher it’ll carry out.
As we confirmed with the Tailgate Index, selecting a desired final result earlier than making any knowledge choices will help in segmenting out essentially the most acceptable coaching and validation info.
Mannequin Growth
With that info useful, we may begin to construct the Tailgate Index.
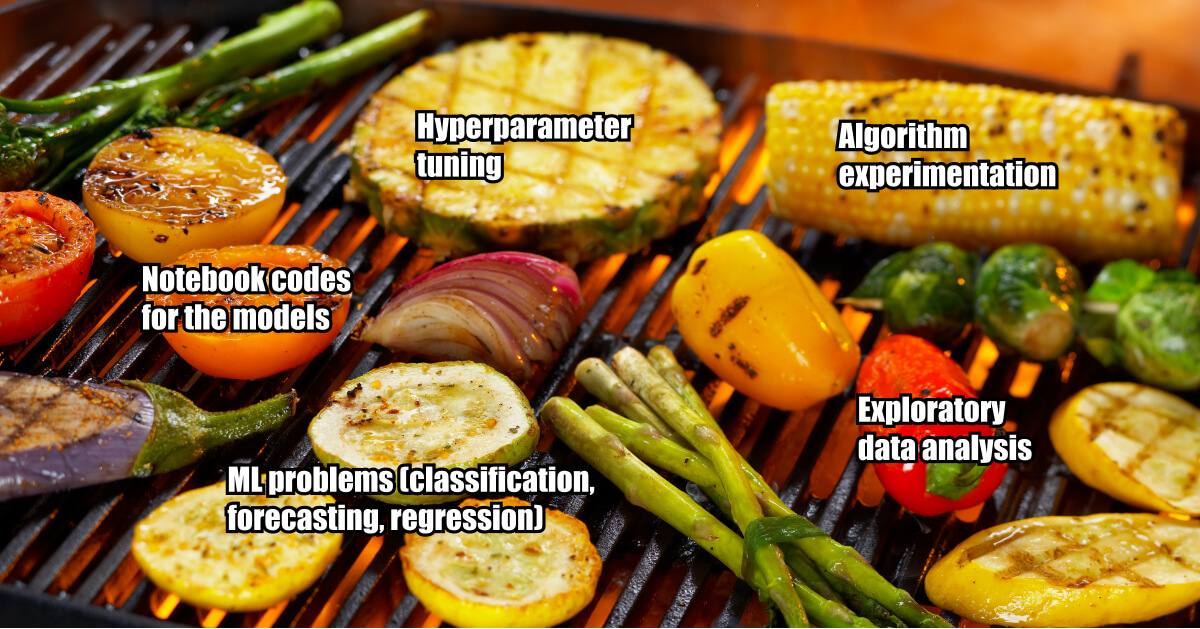
Earlier than constructing the mannequin, we needed to outline the mannequin for the best tailgate day. We labeled a “excellent” day as one the place the climate is between 50 and 80°F, and the cloud cowl is lower than 60%. Then we obtained began.
After manually writing some features of the mannequin, we obtained caught and couldn’t keep in mind some traces of code. As a substitute of toggling back-and-forth between Stack Overflow or scanning tons of Google outcomes, we merely requested Databricks Assistant. With a command in plain English – I would like Python code for a correlation mannequin – Databricks Assistant generated the code, we copied it into our pocket book and shortly added it to the mannequin.
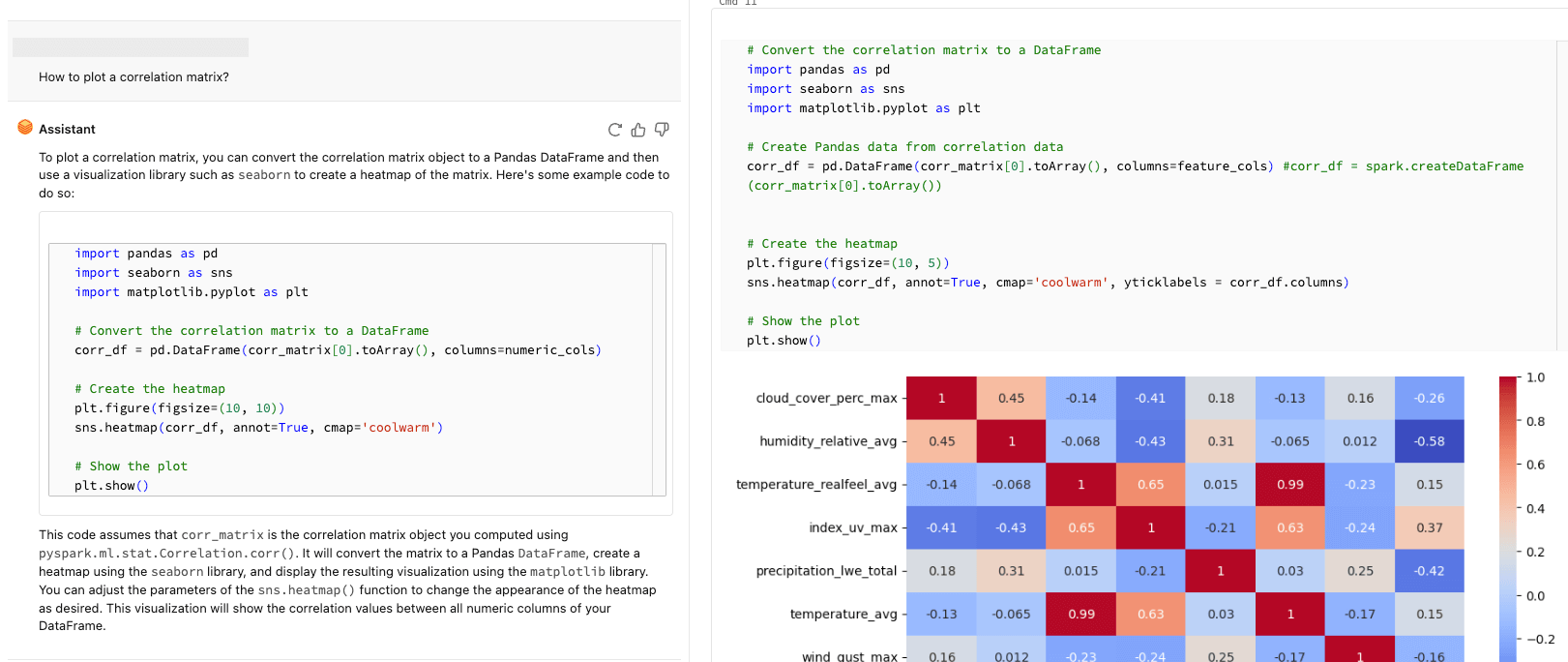
The early iterations of our mannequin had a recall charge – a mirrored image of how precisely it labeled the information we ingested – of roughly 65%. To enhance that, we had to make use of a machine studying approach known as hyperparameter tuning, a course of throughout which we programmatically tweak the mannequin inputs that present the very best outcomes.
Usually, an information scientist can spend hours, days or perhaps weeks altering the parameters of a mannequin to enhance the recall charge. It takes quite a lot of computation and back-end coding. That’s the place AutoML is an enormous assist. Alongside hyperparameter tuning, AutoML will help companies construct totally different ML fashions – like forecasting or regression – with out having to put in writing any code.
For instance, with the Tailgate Index, all we needed to do was load the coaching knowledge into AutoML, and in half-hour, it generated 50 totally different classification fashions for us to select from – all with totally different sensitivity (recall) charges.
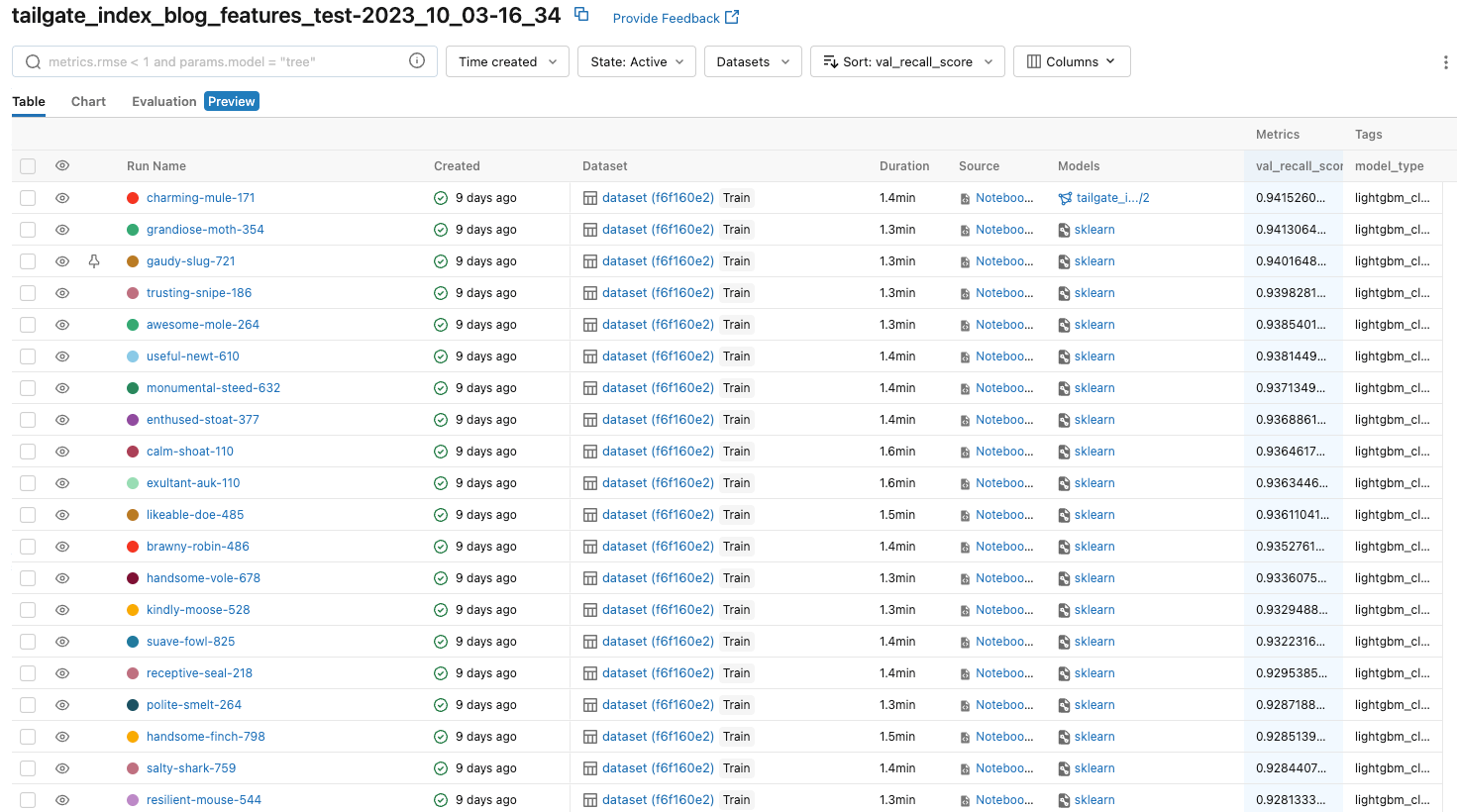
The subsequent step was to decide on a kind of fashions that AutoML offered as our manufacturing mannequin. To simplify this course of, AutoML offers us with a tabular illustration of all mannequin outputs and their corresponding metrics (comparable to sensitivity, specificity, AUC, and so forth.). We sorted these fashions based mostly on sensitivity (recall) to decide on our Tailgate Predictor; a LightGBM Classifier. The ultimate mannequin had a recall charge of 95%. Now, we would have liked to show the mannequin’s consideration from historic knowledge to predicting what’s to return.
To try this, we collected AccuWeather’s forecast knowledge for Nov. 1 to Dec. 31, 2023. Given our goal was to find out best tailgating days, we solely included days with scheduled NFL or faculty soccer video games. AccuWeather additionally had a listing of zip codes which have the NFL and the faculty soccer stadiums, so we have been additionally in a position to filter the information even additional. (Notice: For school soccer, we solely used knowledge associated to the highest 25 groups as of early October.)
So whereas climate forecasts may change, based mostly on the present predictions our mannequin has provide you with the next record of upcoming video games that may be the very best for tailgating.
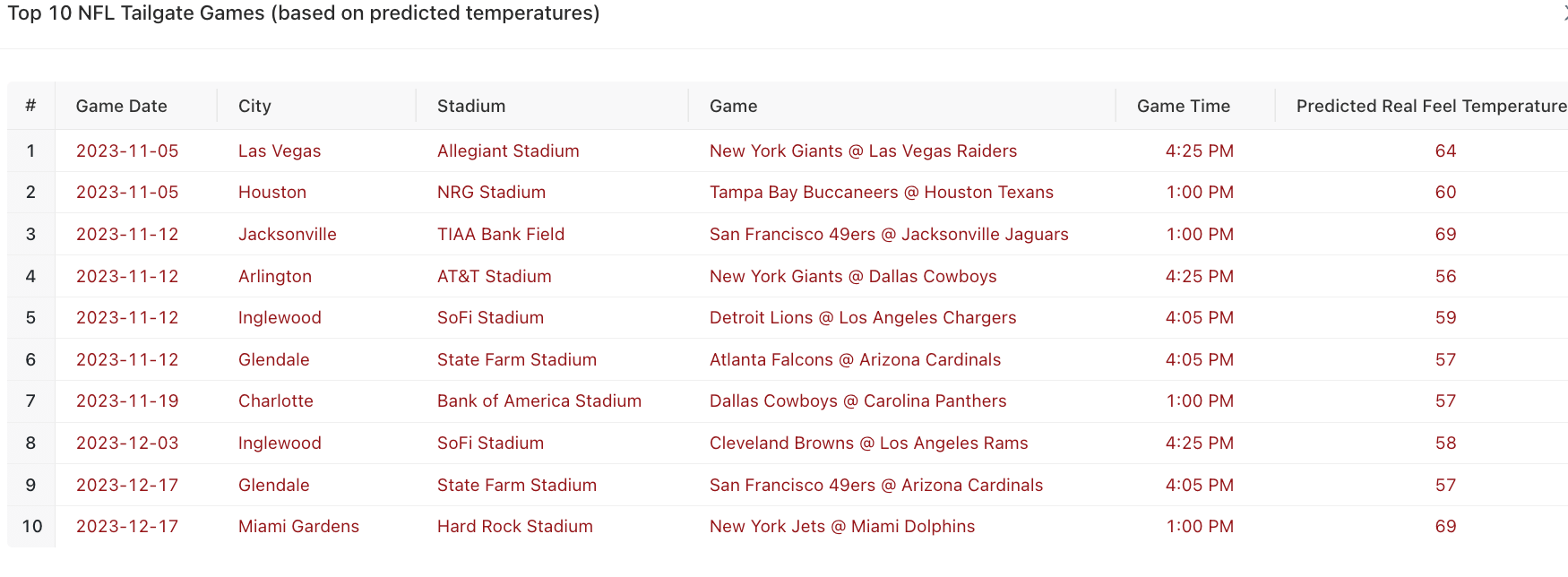
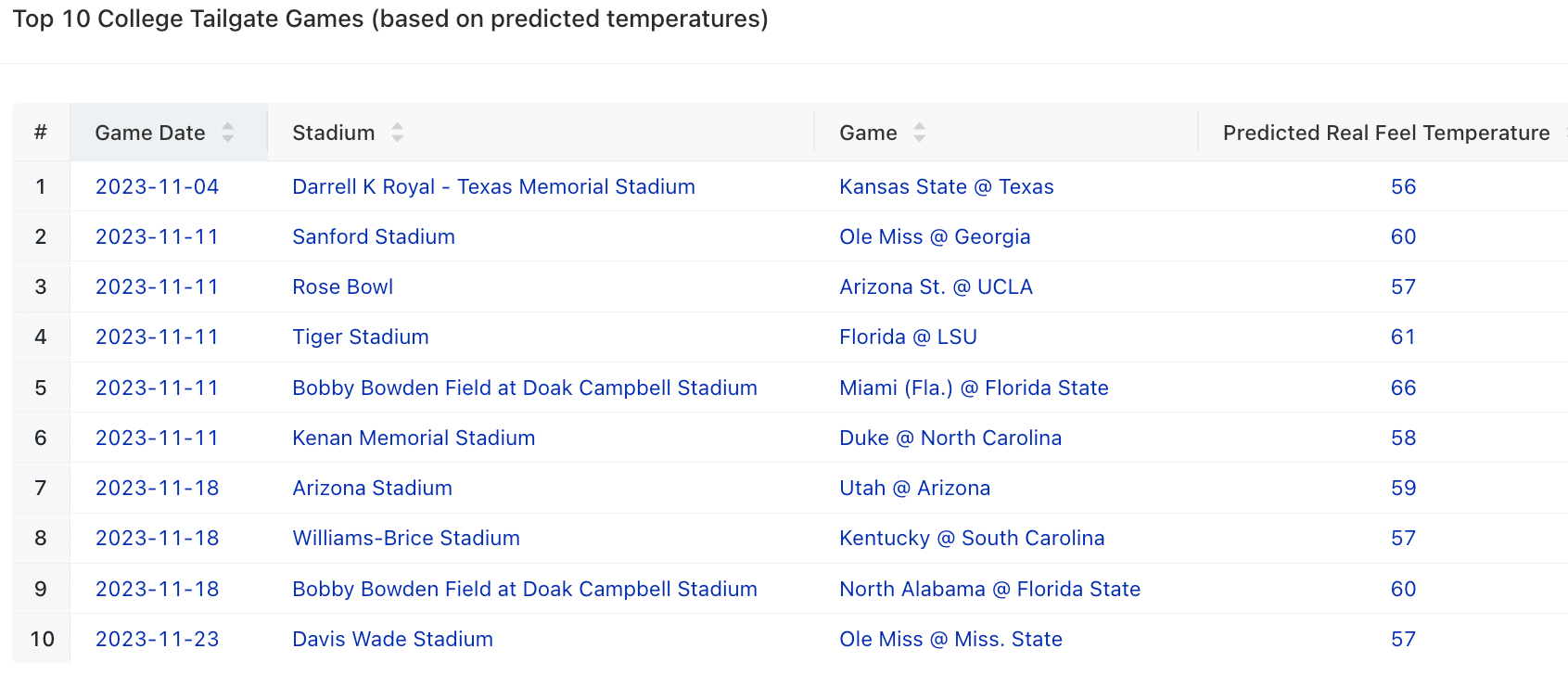
What’s subsequent?
The journey doesn’t finish there. After getting the foundational mannequin proper, we may simply go to Databricks Market and discover further knowledge and AI belongings that may assist customise the mannequin even additional or assist it reply totally different queries.
For companies, one of these flexibility is vital. It’s how firms construct scalable and repeatable AI and ML processes that also present particular person workers the pliability to tailor fashions to their particular issues.
If you happen to’re already utilizing Databricks, head over to the “Machine Studying” part to start out constructing your personal tailgating expertise (or join right here if you wish to give Databricks a strive).
Need to be taught extra about how you should use AccuWeather + Databricks to enhance your backside line? Watch this on-demand session from Information + AI Summit 2023!
[ad_2]